In the era of big data and advanced analytics, understanding statistical significance is crucial for making informed decisions and drawing accurate conclusions. This article delves into the concept of statistical significance, its importance in modern data analysis, and how it can be effectively applied to ensure robust and reliable results.
What is Statistical Significance?
Statistical significance is a measure that helps determine whether the results of a study or experiment are likely to be due to chance or if they reflect a true effect. It provides a way to quantify the likelihood that an observed effect or relationship exists beyond random variation.
In essence, statistical significance assesses the probability that the observed findings are not due to random chance. This is achieved by calculating a p-value, which indicates the probability of obtaining the observed results if the null hypothesis (the assumption that there is no effect or relationship) is true.
Understanding the p-Value
The p-value is central to determining statistical significance. It represents the probability of observing an effect at least as extreme as the one found in your data, assuming the null hypothesis is correct. A smaller p-value suggests that the observed effect is less likely to be due to random chance, thus indicating greater statistical significance.
Traditionally, a p-value threshold of 0.05 has been used as a cutoff for statistical significance. This means that if the p-value is less than 0.05, the results are considered statistically significant, implying a less than 5% chance that the observed effect is due to random variation.
The Role of Statistical Significance in Data Analysis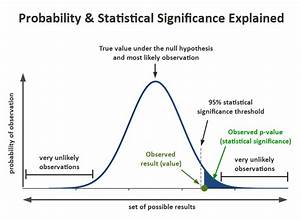
- Guiding Decision-Making: Statistical significance helps analysts and decision-makers determine which results are reliable and actionable. In fields such as medicine, finance, and social sciences, understanding which findings are statistically significant is crucial for making evidence-based decisions.
- Validating Hypotheses: In hypothesis testing, statistical significance helps validate or refute the hypotheses being tested. It provides a measure of confidence that the results support the hypothesis and are not merely a result of random fluctuations in the data.
- Enhancing Research Quality: By adhering to rigorous statistical significance standards, researchers can ensure that their findings are robust and reliable. This contributes to the overall quality of research and helps prevent false positives, where an effect is incorrectly identified as significant.
- Avoiding Misinterpretation: Statistical significance helps avoid misinterpretation of results. Without it, there is a risk of overestimating the importance of findings that may be due to chance rather than a true effect.
Challenges and Misconceptions
- P-Hacking: One challenge associated with statistical significance is the practice of p-hacking, where researchers manipulate data or perform multiple tests to achieve a significant p-value. This can lead to misleading conclusions and false positives.
- Significance vs. Practical Relevance: Statistical significance does not always equate to practical or real-world significance. A result may be statistically significant but not meaningful or relevant in practical terms. It is essential to consider the effect size and its implications for real-world applications.
- Misuse of Thresholds: Relying solely on a p-value threshold (e.g., 0.05) without considering the context of the study can be problematic. It is important to use statistical significance as one component of a broader analysis that includes effect sizes, confidence intervals, and contextual understanding.
Best Practices for Using Statistical Significance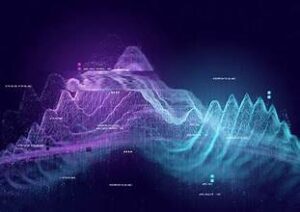
- Combine with Effect Size: Report effect sizes alongside p-values to provide a more comprehensive view of the findings. Effect sizes indicate the magnitude of the observed effect and can help assess its practical significance.
- Use Confidence Intervals: Confidence intervals offer a range within which the true effect is likely to fall. They provide additional context to p-values and help assess the precision and reliability of the results.
- Ensure Proper Study Design: A well-designed study with adequate sample sizes and appropriate methodologies can enhance the reliability of statistical significance. Avoid small sample sizes that can lead to unreliable results and increased risk of Type I and Type II errors.
- Consider Multiple Testing: When conducting multiple tests, apply corrections for multiple comparisons (e.g., Bonferroni correction) to reduce the risk of false positives. This helps ensure that significant findings are not due to random chance alone.
Conclusion
Statistical significance is a fundamental concept in modern data analysis, playing a critical role in validating findings and guiding decision-making. While it provides valuable insights, it should be used in conjunction with other statistical measures and a thorough understanding of the study context. By following best practices and being aware of potential challenges, analysts and researchers can leverage statistical significance to draw accurate and meaningful conclusions from their data.