Statistical Package for the Social Sciences (SPSS) has long been a cornerstone in the field of data analysis. With its robust capabilities and user-friendly interface, it has empowered researchers, analysts, and businesses to extract valuable insights from their data. As the landscape of data analytics evolves, SPSS continues to innovate, introducing new features and enhancements that cater to the growing demands for more advanced data analysis. This article explores the latest innovations in SPSS, highlighting how they can elevate your data analysis capabilities.
1. Enhanced Machine Learning Algorithms
One of the most significant innovations in recent SPSS versions is the enhancement of machine learning algorithms. SPSS now integrates advanced machine learning techniques, such as ensemble methods, neural networks, and deep learning models. These algorithms provide users with more powerful tools for predictive analytics, enabling them to uncover patterns and make data-driven decisions with greater accuracy.
- Ensemble Methods: SPSS has introduced ensemble methods that combine multiple models to improve prediction accuracy. Techniques like Random Forests and Gradient Boosting are now available, allowing users to build more robust models.
- Neural Networks: With the inclusion of neural network capabilities, SPSS users can now apply deep learning techniques to their data, offering greater flexibility and power for complex analyses.
2. Advanced Data Visualization Tools
Data visualization is crucial for interpreting complex data sets, and SPSS has significantly enhanced its visualization capabilities. The latest updates include a range of new and improved visualization tools that help users create more insightful and interactive charts and graphs.
- Customizable Dashboards: SPSS now allows users to create customizable dashboards that aggregate various visualizations into a single view. This feature helps in monitoring key metrics and trends more effectively.
- Interactive Graphs: New interactive graph options enable users to drill down into data points and explore data at a granular level, facilitating a deeper understanding of the results.
3. Integration with Big Data Technologies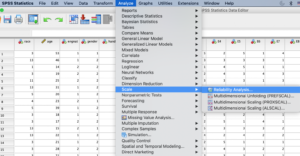
As organizations increasingly adopt big data technologies, SPSS has evolved to integrate seamlessly with these platforms. The latest version of SPSS supports integration with popular big data tools and environments, such as Hadoop and Spark.
- Hadoop Integration: SPSS users can now analyze data stored in Hadoop clusters directly within the SPSS environment, allowing for more efficient handling of large-scale data sets.
- Spark Compatibility: Integration with Apache Spark enables users to leverage distributed computing power for faster and more scalable data analysis.
4. Enhanced Data Management and Preparation
Effective data management and preparation are fundamental to successful data analysis. SPSS has introduced several innovations in this area to streamline data cleaning, transformation, and preparation processes.
- Automated Data Cleaning: The new version includes advanced data cleaning features that automate the identification and correction of inconsistencies, missing values, and outliers.
- Data Transformation Tools: Improved data transformation tools allow users to easily manipulate and reshape data, making it easier to prepare data for analysis.
5. Improved Statistical Testing Capabilities
SPSS continues to enhance its statistical testing capabilities, offering more sophisticated methods for hypothesis testing and data exploration. The latest updates include:
- Robust Statistical Tests: SPSS now provides access to more robust statistical tests, including advanced non-parametric methods and Bayesian statistics, which offer greater flexibility in analyzing complex data sets.
- Advanced Post Hoc Tests: New post hoc testing options allow users to conduct more detailed pairwise comparisons and assess the significance of differences between groups.
6. Enhanced Collaboration Features
In today’s collaborative work environment, the ability to share and collaborate on data analysis projects is essential. SPSS has introduced several new features to facilitate collaboration among team members.
- Shared Workspaces: Users can now create shared workspaces where team members can collaborate on projects, share results, and provide feedback in real-time.
- Version Control: Enhanced version control features track changes and updates to analysis projects, ensuring that teams can manage and review project modifications effectively.
7. Improved User Experience and Interface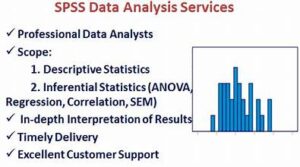
User experience remains a priority for SPSS developers, and the latest updates focus on making the software more intuitive and accessible.
- Streamlined Interface: The SPSS interface has been redesigned for greater usability, with a more intuitive layout and improved navigation.
- Enhanced Help Resources: New help resources and tutorials are available within the software, providing users with on-demand support and guidance.
Conclusion
The latest innovations in SPSS represent significant advancements in the field of data analysis, offering enhanced machine learning algorithms, advanced data visualization tools, and seamless integration with big data technologies. These updates empower users to conduct more sophisticated analyses, manage and prepare data more efficiently, and collaborate more effectively. As data continues to drive decision-making in various sectors, staying up-to-date with these innovations will be crucial for leveraging the full potential of SPSS.