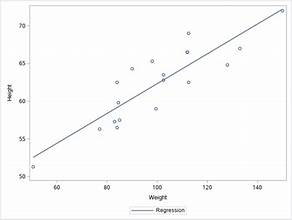
In the realm of data analysis, regression analysis stands out as a powerful tool for predicting outcomes based on various input variables. At the heart of regression analysis are regression coefficients, which quantify the relationship between predictors and the outcome variable. Optimizing these coefficients is crucial for ensuring accurate and reliable predictions. This article will explore best practices for working with regression coefficients to enhance your statistical analysis.
Understanding Regression Coefficients
Regression coefficients are numerical values that represent the relationship between independent variables (predictors) and the dependent variable (outcome). In a simple linear regression model, the coefficient measures the change in the outcome variable for a one-unit change in the predictor variable. In multiple regression models, coefficients indicate the impact of each predictor while holding other predictors constant.
Best Practices for Optimizing Regression Coefficients
1. Select Relevant Variables
Before diving into coefficient optimization, ensure that you are using relevant predictors. Including irrelevant variables can lead to overfitting, where the model performs well on training data but poorly on new data. Use domain knowledge and statistical methods such as correlation analysis or feature selection techniques to identify variables that have a significant relationship with the outcome.
2. Standardize Your Variables
Standardizing variables (i.e., transforming them to have a mean of zero and a standard deviation of one) can make interpretation of regression coefficients easier, especially when dealing with predictors measured on different scales. Standardization also helps in comparing the relative importance of predictors.
3. Check for Multicollinearity
Multicollinearity occurs when predictor variables are highly correlated with each other. This can inflate the standard errors of the coefficients and make it difficult to assess the individual effect of each predictor. Use variance inflation factor (VIF) to detect multicollinearity and consider removing or combining correlated predictors to mitigate its effects.
4. Regularize Your Model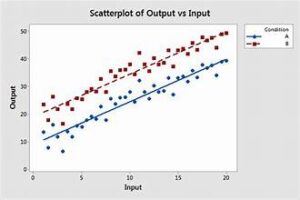
Regularization techniques, such as Ridge and Lasso regression, help in controlling the complexity of the model by penalizing large coefficients. Ridge regression adds a penalty proportional to the square of the coefficients, while Lasso regression adds a penalty proportional to the absolute values of the coefficients. These techniques can improve model performance and reduce overfitting.
5. Assess and Validate Your Model
After fitting your regression model, it’s essential to evaluate its performance. Use metrics such as R-squared, adjusted R-squared, and mean squared error (MSE) to assess the model’s goodness-of-fit. Additionally, perform cross-validation to test the model’s performance on unseen data, ensuring that your coefficients are robust and not just tailored to the training data.
6. Interpret Coefficients Carefully
Interpreting regression coefficients requires careful consideration of the context and scale of the variables. A coefficient indicates the expected change in the outcome variable for a one-unit change in the predictor. However, the practical significance of this change should be evaluated in the context of the problem being studied.
7. Update Your Model Regularly
In dynamic environments where data and relationships between variables may change over time, it’s crucial to update your regression model periodically. Re-assessing and recalibrating the coefficients based on the most recent data helps maintain the accuracy and relevance of your predictions.
Conclusion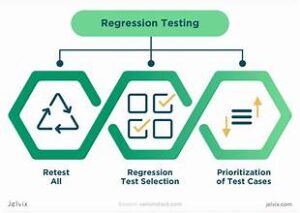
Optimizing regression coefficients is an integral part of enhancing the accuracy and reliability of statistical analysis. By selecting relevant variables, standardizing data, addressing multicollinearity, applying regularization, and validating your model, you can ensure that your regression analysis provides meaningful and actionable insights. Remember that accurate predictions are the result of a well-constructed model and careful interpretation of the coefficients. Embrace these best practices to maximize the effectiveness of your regression analysis and drive better decision-making.