In the ever-evolving world of data analysis, hypothesis testing remains a cornerstone for drawing meaningful conclusions from empirical data. As we navigate through 2024, advancements in technology and methodology continue to reshape how we approach and execute hypothesis testing. This comprehensive guide explores the latest techniques and trends in hypothesis testing, ensuring that you stay ahead in the data analysis game.
Understanding Hypothesis Testing
Hypothesis testing is a statistical method used to make inferences or draw conclusions about a population based on sample data. It involves formulating two competing hypotheses:
- Null Hypothesis (H0): This hypothesis states that there is no effect or no difference, and it is the default assumption that any observed difference is due to random chance.
- Alternative Hypothesis (H1 or Ha): This hypothesis asserts that there is an effect or a difference, challenging the null hypothesis.
The goal of hypothesis testing is to determine whether there is enough statistical evidence to reject the null hypothesis in favor of the alternative hypothesis.
Latest Techniques in Hypothesis Testing for 2024
1. Bayesian Hypothesis Testing
Bayesian methods have gained prominence in recent years due to their flexibility and the ability to incorporate prior knowledge into the analysis. In Bayesian hypothesis testing, prior distributions are updated with observed data to provide posterior distributions. This approach offers a probabilistic framework for hypothesis testing, allowing for a more nuanced understanding of evidence and uncertainty.
Key Advantages:
- Incorporates Prior Knowledge: Useful when historical data or expert opinion is available.
- Probabilistic Interpretation: Provides a direct probability of the hypothesis being true, as opposed to p-values.
2. Machine Learning Integration
Machine learning techniques are increasingly being integrated with traditional hypothesis testing methods to enhance predictive accuracy and pattern recognition. Techniques such as automated feature selection and model tuning can improve the robustness of hypothesis tests by identifying relevant variables and interactions.
Key Advantages:
- Enhanced Accuracy: Machine learning algorithms can identify complex patterns that traditional methods might miss.
- Automation: Reduces manual intervention and accelerates the hypothesis testing process.
3. Permutation Tests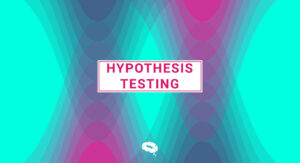
Permutation tests, also known as randomization tests, have gained traction due to their non-parametric nature. They involve repeatedly shuffling the data and recalculating the test statistic to build a distribution under the null hypothesis. This method is particularly useful when assumptions of traditional tests (e.g., normality) are violated.
Key Advantages:
- Flexibility: Does not rely on parametric assumptions.
- Applicability: Useful for small sample sizes or non-standard test statistics.
4. False Discovery Rate (FDR) Control
The False Discovery Rate (FDR) is a crucial concept in multiple hypothesis testing. As the number of hypotheses increases, the chance of incorrectly rejecting the null hypothesis (Type I error) also increases. FDR control methods, such as the Benjamini-Hochberg procedure, help manage the proportion of false positives among rejected hypotheses.
Key Advantages:
- Multiple Testing Correction: Reduces the likelihood of false positives in large-scale testing scenarios.
- Adjustable: Allows for a balance between discovering true effects and controlling false discoveries.
Trends in Hypothesis Testing for 2024
1. Emphasis on Reproducibility and Transparency
The reproducibility crisis in science has highlighted the need for transparent and reproducible research practices. In 2024, there is a strong emphasis on ensuring that hypothesis testing procedures are well-documented and reproducible. This trend includes sharing data, code, and methodologies openly to facilitate verification and validation of results.
Key Practices:
- Open Data: Sharing datasets publicly to allow for independent verification.
- Reproducible Code: Providing code and detailed methodologies to ensure that results can be replicated.
2. Focus on Data Ethics and Privacy
As data privacy concerns grow, ethical considerations in data analysis are becoming increasingly important. Hypothesis testing in 2024 places a strong emphasis on adhering to ethical standards and protecting participant privacy. Techniques such as differential privacy are being incorporated to ensure that individual data points cannot be re-identified.
Key Practices:
- Differential Privacy: Adding noise to data to protect individual privacy.
- Ethical Guidelines: Adhering to ethical standards in data collection and analysis.
3. Incorporation of Big Data
The rise of big data has transformed hypothesis testing by providing vast amounts of information for analysis. Techniques are evolving to handle the complexity and volume of big data, including distributed computing and advanced data management strategies.
Key Practices:
- Scalable Algorithms: Using algorithms designed to handle large-scale data efficiently.
- Data Management: Implementing robust data management practices to handle diverse and voluminous data.
4. Cross-Disciplinary Approaches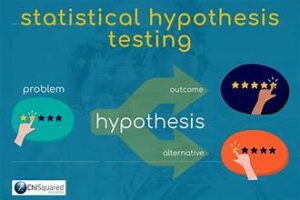
Hypothesis testing is increasingly adopting cross-disciplinary approaches, integrating knowledge and techniques from various fields such as genomics, economics, and social sciences. This trend allows for more comprehensive analyses and insights across different domains.
Key Practices:
- Interdisciplinary Collaboration: Collaborating with experts from different fields to enhance analysis.
- Diverse Methodologies: Applying diverse methodologies to address complex research questions.
Best Practices for Effective Hypothesis Testing
To effectively apply these techniques and trends in hypothesis testing, consider the following best practices:
- Clearly Define Hypotheses: Ensure that both null and alternative hypotheses are clearly stated and well-defined.
- Choose Appropriate Methods: Select the testing methods that best fit the data and research question.
- Validate Assumptions: Check assumptions underlying the chosen methods and use alternative methods if necessary.
- Report Results Transparently: Present results clearly, including p-values, effect sizes, and confidence intervals.
- Adopt Reproducibility Measures: Document and share data and code to enable replication of results.
Conclusion
Hypothesis testing remains a fundamental tool in data analysis, and staying updated with the latest techniques and trends is crucial for effective research. As we progress through 2024, the integration of Bayesian methods, machine learning, permutation tests, and FDR control, combined with a focus on reproducibility, ethics, big data, and cross-disciplinary approaches, will shape the future of hypothesis testing. By adhering to best practices and embracing these advancements, you can enhance the robustness and credibility of your data analysis endeavors.