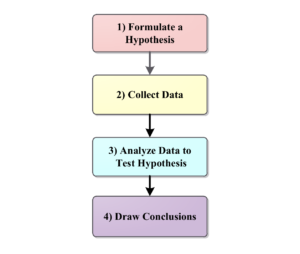
In the dynamic landscape of data science, 2024 marks a significant turning point with the advent of advanced hypothesis testing techniques. As organizations increasingly rely on data-driven decisions, the need for robust, precise, and innovative methods to validate hypotheses has never been more critical. This article delves into the transformative impact of these advanced techniques on data science, highlighting their benefits, applications, and the future they herald.
Understanding Hypothesis Testing
Hypothesis testing is a fundamental aspect of statistical analysis in data science. It involves making an assumption (the hypothesis) about a population parameter and then using sample data to test this assumption. Traditional methods like t-tests, chi-square tests, and ANOVA have been the bedrock of hypothesis testing. However, the complexity and volume of modern data demand more sophisticated approaches.
The Evolution of Hypothesis Testing Techniques
- Bayesian Hypothesis Testing
Bayesian methods are becoming increasingly popular due to their flexibility and the intuitive way they handle uncertainty. Unlike classical methods, Bayesian hypothesis testing incorporates prior knowledge and updates the probability of a hypothesis as more data becomes available. This approach allows data scientists to make more informed decisions, especially in cases with limited data.
- Permutation Tests
Permutation tests are non-parametric methods that do not rely on the assumption of a specific distribution. They involve rearranging the data and calculating the test statistic for each arrangement to build a distribution of the test statistic under the null hypothesis. This technique is particularly useful in complex datasets where traditional parametric methods might fail.
- Bootstrap Methods
Bootstrapping is a resampling technique that provides a way to estimate the sampling distribution of a statistic by repeatedly resampling with replacement from the data. This method is valuable for estimating the confidence intervals and significance tests for complex models that traditional methods cannot handle.
The Impact of Advanced Hypothesis Testing on Data Science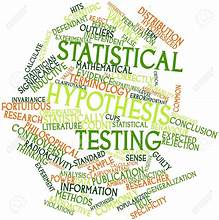
- Improved Decision-Making
Advanced hypothesis testing techniques offer more precise and reliable results, enabling organizations to make better data-driven decisions. By incorporating these methods, data scientists can reduce the risk of Type I and Type II errors, leading to more accurate and actionable insights.
- Handling Big Data
The sheer volume and variety of data in today’s world require methods that can scale efficiently. Advanced techniques such as permutation tests and bootstrapping are well-suited for big data environments, allowing for more robust analysis and interpretation of large datasets.
- Enhanced Model Validation
In machine learning, model validation is crucial to ensure that models generalize well to new data. Advanced hypothesis testing provides more rigorous ways to assess model performance, leading to more reliable and trustworthy models.
Applications in Various Fields
- Healthcare
In healthcare, precise data analysis can save lives. Advanced hypothesis testing techniques are used to analyze clinical trial data, identify significant biomarkers, and validate predictive models for disease diagnosis and treatment.
- Finance
In the financial sector, these techniques help in risk assessment, fraud detection, and investment strategy validation. By providing more accurate analysis, financial institutions can make better-informed decisions, minimize risks, and optimize returns.
- Marketing
Marketing analytics benefits from advanced hypothesis testing by enabling more precise targeting and segmentation. Marketers can validate campaign effectiveness, understand consumer behavior, and optimize marketing strategies with greater confidence.
The Future of Hypothesis Testing in Data Science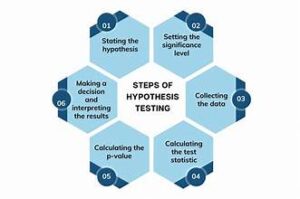
As we look to the future, the integration of machine learning with hypothesis testing will play a pivotal role. Automated hypothesis generation and testing using AI will streamline the analytical process, allowing data scientists to focus on interpreting results and deriving strategic insights.
Moreover, the development of new statistical methods and the continuous improvement of existing ones will ensure that hypothesis testing keeps pace with the evolving demands of data science. Collaborative efforts between statisticians, data scientists, and domain experts will drive innovation, leading to more advanced, robust, and versatile hypothesis testing techniques.
Conclusion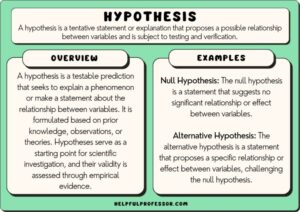
In 2024, advanced hypothesis testing techniques are revolutionizing data science by providing more accurate, reliable, and scalable methods for data analysis. As these techniques continue to evolve, they will unlock new possibilities across various fields, driving better decision-making and fostering innovation. Embracing these advancements is crucial for organizations aiming to leverage the full potential of their data and stay ahead in an increasingly data-driven world.