In the age of big data, businesses are increasingly relying on predictive analytics to make informed decisions. One of the most powerful tools in the predictive analytics toolbox is the linear regression model. This article explores how linear regression models unlock data insights and drive strategic decision-making in various industries.
Understanding Linear Regression
Linear regression is a statistical method used to model the relationship between a dependent variable and one or more independent variables. The primary objective is to predict the dependent variable based on the values of the independent variables. The relationship is modeled through a linear equation:
y=β0+β1×1+β2×2+…+βnxn+ϵy = \beta_0 + \beta_1x_1 + \beta_2x_2 + … + \beta_nx_n + \epsilony=β0+β1x1+β2x2+…+βnxn+ϵ
Where:
- yyy is the dependent variable.
- β0\beta_0β0 is the intercept.
- β1,β2,…,βn\beta_1, \beta_2, …, \beta_nβ1,β2,…,βn are the coefficients of the independent variables.
- x1,x2,…,xnx_1, x_2, …, x_nx1,x2,…,xn are the independent variables.
- ϵ\epsilonϵ is the error term.
The Power of Linear Regression in Predictive Analytics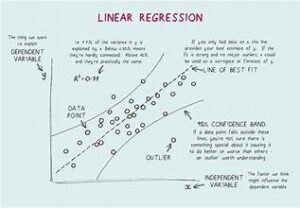
1. Simplicity and Interpretability
Linear regression models are straightforward and easy to interpret. The coefficients indicate the strength and direction of the relationship between each independent variable and the dependent variable. This simplicity makes linear regression an attractive choice for analysts and decision-makers who need clear and actionable insights.
2. Efficiency in Predictive Performance
Despite its simplicity, linear regression can be highly effective in predicting outcomes. It works well with linear relationships and can be extended to capture non-linear patterns using polynomial regression or interaction terms. For many applications, the predictive performance of linear regression is on par with more complex models.
3. Identifying Key Drivers
Linear regression helps identify the key drivers behind an outcome. By analyzing the coefficients, businesses can determine which factors significantly impact their metrics. This knowledge allows for targeted interventions and resource allocation, optimizing performance and efficiency.
4. Versatility Across Industries
Linear regression is used across various industries, from finance to healthcare. In finance, it can predict stock prices and assess credit risk. In healthcare, it helps in predicting patient outcomes and optimizing treatment plans. Its versatility makes it a go-to model for many predictive analytics tasks.
Building a Linear Regression Model: A Step-by-Step Guide
Step 1: Data Collection and Preparation
The first step in building a linear regression model is collecting and preparing the data. This involves:
- Gathering relevant data: Ensure the data includes all potential independent variables.
- Cleaning the data: Handle missing values, outliers, and inconsistencies.
- Feature selection: Choose the most relevant variables to include in the model.
Step 2: Splitting the Data
Split the data into training and testing sets. The training set is used to build the model, while the testing set evaluates its performance. A common practice is to use 70-80% of the data for training and the remaining 20-30% for testing.
Step 3: Model Training
Train the linear regression model using the training data. This involves estimating the coefficients (β\betaβ) that minimize the difference between the predicted and actual values of the dependent variable.
Step 4: Model Evaluation
Evaluate the model’s performance using the testing data. Common metrics include:
- R-squared: Measures the proportion of variance in the dependent variable explained by the independent variables.
- Mean Squared Error (MSE): Calculates the average squared difference between predicted and actual values.
- Root Mean Squared Error (RMSE): The square root of MSE, providing a measure in the same units as the dependent variable.
Step 5: Model Optimization
Optimize the model by tweaking parameters, adding interaction terms, or applying regularization techniques like Lasso or Ridge regression to prevent overfitting and improve predictive performance.
Real-World Applications of Linear Regression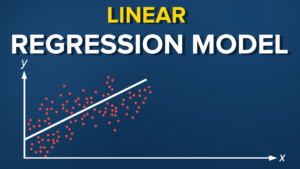
1. Marketing and Sales
Linear regression models help marketers predict customer behavior, optimize pricing strategies, and improve campaign effectiveness. By understanding the factors that drive sales, businesses can tailor their marketing efforts to target the right audience at the right time.
2. Finance
In finance, linear regression is used to forecast economic indicators, predict stock prices, and assess credit risk. It enables financial institutions to make data-driven decisions, manage risk, and maximize returns.
3. Healthcare
Healthcare providers use linear regression to predict patient outcomes, optimize treatment plans, and allocate resources efficiently. By analyzing patient data, hospitals can improve care quality and reduce costs.
4. Manufacturing
Manufacturers apply linear regression to optimize production processes, forecast demand, and reduce waste. It helps in identifying the key factors affecting production efficiency and product quality.
Conclusion
Linear regression models are a cornerstone of predictive analytics, offering simplicity, interpretability, and versatility. By unlocking data insights, these models empower businesses to make informed decisions, optimize performance, and stay competitive in an increasingly data-driven world.
Implementing linear regression in your predictive analytics strategy can drive significant improvements across various domains. Embrace the power of linear regression and transform your data into actionable insights for sustained success.