In the realm of modern data analytics, Bayes’ Theorem stands out as a fundamental concept, shaping how data is interpreted and decisions are made. This guide will delve into the impact of Bayes’ Theorem on contemporary data analysis, illustrating its importance, applications, and how it enhances the precision and relevance of insights derived from data.
What is Bayes’ Theorem?
Bayes’ Theorem is a mathematical formula used to determine the probability of a given event based on prior knowledge of conditions related to the event. Named after the Reverend Thomas Bayes, the theorem provides a way to update the probability of a hypothesis as more evidence or information becomes available.
The theorem is expressed as:
P(A∣B)=P(B∣A)⋅P(A)P(B)P(A|B) = \frac{P(B|A) \cdot P(A)}{P(B)}P(A∣B)=P(B)P(B∣A)⋅P(A)
Where:
- P(A∣B)P(A|B)P(A∣B) is the posterior probability of event A given that B has occurred.
- P(B∣A)P(B|A)P(B∣A) is the likelihood of event B given that A is true.
- P(A)P(A)P(A) is the prior probability of event A.
- P(B)P(B)P(B) is the marginal probability of event B.
The Relevance of Bayes’ Theorem in Data Analytics
Bayes’ Theorem revolutionizes data analytics by allowing analysts to incorporate prior knowledge and continuously update probabilities as new data emerges. This approach enhances decision-making and predictive modeling in various domains. Here’s how Bayes’ Theorem impacts modern data analytics:
1. Improved Predictive Modeling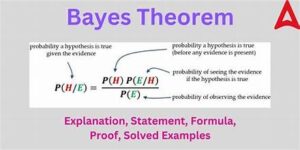
In predictive analytics, Bayes’ Theorem enables the development of models that can forecast future events with higher accuracy. By combining historical data with prior probabilities, analysts can refine their predictions and adapt to changing conditions. For instance, in customer churn prediction, Bayes’ Theorem helps in estimating the likelihood of a customer leaving based on past behaviors and demographic data.
2. Enhanced Risk Assessment
Risk management benefits greatly from Bayes’ Theorem. It allows businesses to assess risks more precisely by incorporating both prior information and new evidence. For example, in financial risk assessment, analysts use Bayes’ Theorem to update the probability of default on loans based on recent economic indicators and borrower history.
3. Optimized Decision-Making
Bayes’ Theorem facilitates better decision-making by providing a structured approach to handle uncertainty. In sectors like healthcare, it helps in diagnosing diseases by combining prior knowledge about the prevalence of conditions with new test results. This method enhances the accuracy of diagnoses and treatment plans.
4. Advanced Fraud Detection
Fraud detection systems use Bayes’ Theorem to identify suspicious activities by continuously updating the probability of fraudulent behavior based on new transaction data. This approach improves the efficiency of detecting anomalies and reduces false positives.
5. Refined Marketing Strategies
In marketing, Bayes’ Theorem helps in segmenting customers and personalizing offers. By analyzing past interactions and customer behavior, marketers can update their understanding of customer preferences and tailor campaigns to target specific segments more effectively.
Applications of Bayes’ Theorem in Data Analytics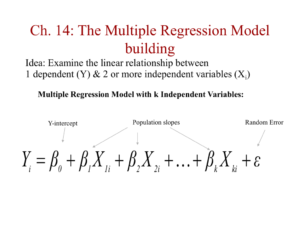
1. Spam Filtering
One of the most common applications of Bayes’ Theorem is in spam filtering. Email services use Bayesian filters to classify emails as spam or not spam based on the likelihood of certain words or phrases appearing in spam messages.
2. Medical Diagnosis
Bayesian methods are widely used in medical diagnostics to update the probability of a disease given new symptoms or test results. This approach provides a more accurate diagnosis by integrating prior knowledge with current evidence.
3. Financial Forecasting
Bayesian models are employed in financial forecasting to predict market trends and asset prices. By continuously updating predictions with new market data, analysts can make more informed investment decisions.
4. Natural Language Processing (NLP)
In NLP, Bayes’ Theorem aids in text classification tasks such as sentiment analysis and topic modeling. Bayesian methods help in understanding the context and intent behind textual data, improving the accuracy of language models.
Benefits of Using Bayes’ Theorem
- Adaptability: Bayes’ Theorem allows models to adapt to new information, improving their accuracy over time.
- Handling Uncertainty: It provides a systematic approach to deal with uncertainty and incomplete information.
- Integration of Prior Knowledge: The theorem leverages existing knowledge, making it easier to build robust models with limited data.
Conclusion
Bayes’ Theorem plays a pivotal role in modern data analytics by enhancing predictive modeling, risk assessment, decision-making, fraud detection, and marketing strategies. Its ability to incorporate prior knowledge and update probabilities with new evidence makes it an indispensable tool in the data analyst’s toolkit. As data continues to grow in complexity and volume, the application of Bayes’ Theorem will likely become even more integral to deriving meaningful insights and making informed decisions.