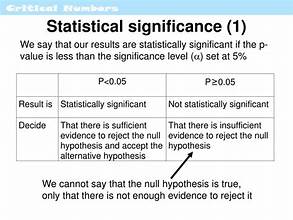
In the realm of research and data analysis, statistical significance is a cornerstone concept that influences the interpretation of results and subsequent decision-making. This article will explore what statistical significance is, why it matters, and how it impacts research outcomes and decision-making processes.
What is Statistical Significance?
Statistical significance is a measure used to determine whether the results of a study are likely to be due to chance or if they reflect a true effect. It is typically assessed through hypothesis testing, where researchers test an initial assumption (null hypothesis) against an alternative hypothesis. The result is quantified using a p-value, which indicates the probability of obtaining the observed results if the null hypothesis were true.
Key Definitions:
- Null Hypothesis (H0): The default position that there is no effect or no difference.
- Alternative Hypothesis (H1): The position that there is an effect or a difference.
- P-value: The probability of observing the test results under the null hypothesis. A p-value less than the chosen significance level (often 0.05) indicates statistical significance.
Why Statistical Significance Matters
Statistical significance provides a framework for evaluating the validity of research findings. Here are a few reasons why it is crucial:
- Validity of Results: Statistical significance helps determine whether the observed effects in a study are genuine or if they occurred by random chance. This helps in validating the research findings.
- Decision-Making: In fields such as medicine, social sciences, and economics, statistically significant results can influence policy decisions, medical treatments, and business strategies. Accurate significance testing ensures that these decisions are based on reliable evidence.
- Resource Allocation: Organizations often use statistical significance to justify the allocation of resources. For instance, a significant finding might lead to further investment in a particular area of research.
How Statistical Significance Impacts Research Outcomes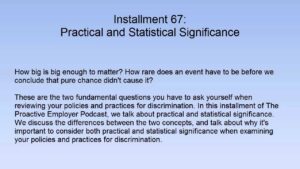
- Interpreting Results:
- Significant Results: A statistically significant result suggests that the observed effect is unlikely to have occurred by chance. For example, if a new drug shows significant improvement in patient outcomes compared to a placebo, it may be considered effective.
- Non-Significant Results: If a result is not statistically significant, it means there is insufficient evidence to reject the null hypothesis. This does not prove that there is no effect, but rather that the evidence is not strong enough to support it.
- Influence on Research Direction:
- Publication Bias: Significant results are more likely to be published, which can lead to publication bias. Researchers and journals may favor studies with significant findings, potentially skewing the overall scientific literature.
- Future Research: Significant results can pave the way for further research, while non-significant findings might prompt researchers to reconsider their hypotheses or methods.
Factors Affecting Statistical Significance
- Sample Size: Larger sample sizes generally provide more accurate estimates of the population parameters and increase the likelihood of detecting a true effect. Small sample sizes can lead to less reliable results and higher variability.
- Effect Size: The magnitude of the effect observed in a study also plays a role. Even a small effect can be statistically significant if the sample size is large enough, while a large effect might not be significant if the sample size is too small.
- Significance Level (α): The threshold for statistical significance (commonly set at 0.05) is the probability of rejecting the null hypothesis when it is actually true (Type I error). Researchers must choose an appropriate significance level based on the context of their study.
Common Misconceptions About Statistical Significance
- Statistical Significance vs. Practical Significance: A result can be statistically significant but not practically meaningful. For example, a study may find a statistically significant difference in test scores between two teaching methods, but if the difference is minimal, it may not have practical implications.
- P-value Misinterpretation: A p-value is not the probability that the null hypothesis is true, nor does it measure the size of the effect. It only indicates the likelihood of observing the results given that the null hypothesis is true.
Statistical Significance and Decision-Making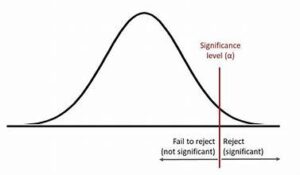
- Policy and Practice: In policy-making, statistically significant findings can drive changes in laws or regulations. For instance, a study showing a significant correlation between air pollution and health issues might lead to stricter environmental regulations.
- Business Strategies: Companies use statistical significance to evaluate marketing campaigns, product performance, and customer satisfaction. Significant results can lead to strategic decisions that enhance business outcomes.
- Medical Interventions: In medicine, clinical trials rely on statistical significance to determine the efficacy of treatments. A statistically significant result can lead to the approval of new drugs or medical procedures.
Conclusion
Understanding statistical significance is essential for interpreting research findings and making informed decisions. By providing a measure of whether observed effects are likely due to chance, statistical significance helps ensure that decisions are based on reliable evidence. However, it is crucial to consider other factors, such as effect size and practical implications, to fully understand the impact of research outcomes.