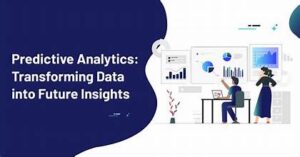
In the realm of predictive analytics, random variables play a crucial role in modeling uncertainty and variability. Understanding their impact is essential for developing robust models that provide accurate forecasts and actionable insights. This article delves into the significance of random variables in predictive analytics, explores current trends, and anticipates future developments.
What Are Random Variables?
A random variable is a numerical outcome of a random phenomenon. In statistical terms, it is a function that assigns a real number to each possible outcome of a random experiment. There are two main types of random variables:
- Discrete Random Variables: These take on countable values, such as the number of goals scored in a football match.
- Continuous Random Variables: These can assume any value within a given range, such as the temperature in a city over time.
The Role of Random Variables in Predictive Analytics
Predictive analytics involves using historical data to make informed predictions about future events. Random variables are integral to this process as they help quantify uncertainty and model complex phenomena. Here’s how they impact predictive analytics:
1. Modeling Uncertainty
Random variables allow analysts to model uncertainty and variability in data. For instance, when predicting stock prices, analysts use random variables to account for market fluctuations and other unpredictable factors. This helps in creating models that can provide a range of possible outcomes rather than a single deterministic value.
2. Improving Model Accuracy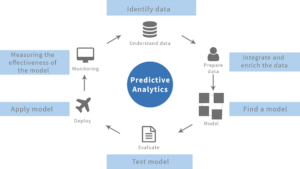
By incorporating random variables, predictive models can better capture the underlying data patterns. Techniques such as Monte Carlo simulations use random variables to generate multiple scenarios and assess the likelihood of different outcomes. This enhances the accuracy of predictions by considering a wide array of possible future states.
3. Risk Assessment
Predictive models that utilize random variables are crucial for risk assessment. In financial sectors, for instance, models that account for random variables can estimate the probability of extreme losses or gains. This helps in making informed decisions and developing strategies to mitigate potential risks.
Current Trends in Predictive Analytics
The use of random variables in predictive analytics is evolving with advancements in technology and methodology. Here are some key trends:
1. Integration with Machine Learning
Machine learning algorithms increasingly incorporate random variables to improve predictive performance. Techniques such as Bayesian inference and probabilistic modeling use random variables to update predictions as new data becomes available. This integration enhances the ability of models to adapt and provide more accurate forecasts.
2. Big Data Analytics
The rise of big data has transformed predictive analytics. With vast amounts of data being generated, models now leverage random variables to handle complex datasets and identify patterns that were previously undetectable. This helps in making predictions with higher precision and relevance.
3. Real-Time Analytics
Real-time predictive analytics is becoming more prevalent. Random variables are used to process streaming data and provide instant insights. For example, in online marketing, random variables help in predicting customer behavior in real-time, allowing businesses to make timely adjustments to their strategies.
Future Trends in Predictive Analytics
As technology advances, the role of random variables in predictive analytics is likely to become even more sophisticated. Here are some future trends to watch:
1. Enhanced Algorithms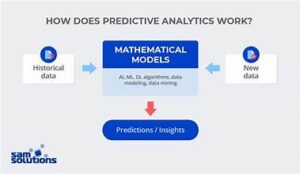
Future predictive models will continue to refine the use of random variables through advanced algorithms. Techniques such as deep learning and neural networks will integrate random variables more effectively to capture intricate patterns and improve prediction accuracy.
2. Quantum Computing
Quantum computing holds the potential to revolutionize predictive analytics. By leveraging quantum algorithms, future models could handle complex random variables more efficiently, providing faster and more accurate predictions for large-scale problems.
3. Ethical Considerations
As predictive analytics becomes more influential, ethical considerations regarding the use of random variables will gain importance. Ensuring that models are fair and unbiased, and addressing privacy concerns, will be crucial in the development and application of predictive analytics.
Conclusion
Random variables are fundamental to predictive analytics, enabling the modeling of uncertainty, improving model accuracy, and assessing risk. As technology advances, their role will continue to evolve, with trends such as machine learning integration, big data analytics, and real-time processing shaping the future of predictive analytics. Staying informed about these developments will be key for leveraging random variables effectively in predictive models and making data-driven decisions.
Understanding the impact of random variables on predictive analytics helps in harnessing their full potential and preparing for future advancements. By embracing these insights and trends, businesses and analysts can enhance their predictive capabilities and drive better outcomes in an increasingly complex and data-driven world.