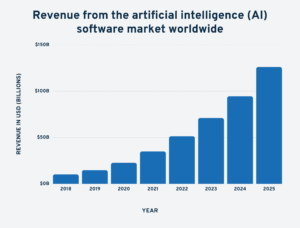
Introduction
In the evolving world of finance, artificial intelligence (AI) is transforming how market predictions are made. By leveraging advanced algorithms and machine learning models, AI can analyze vast amounts of data to forecast financial trends with unprecedented accuracy. However, the integration of AI into financial market predictions also brings up crucial statistical concepts such as P-values and statistical significance. This article delves into how AI impacts financial market predictions and the importance of understanding P-values and statistical significance in this context.
The Role of AI in Financial Market Predictions
AI has become a pivotal tool in financial markets, enhancing predictive models and investment strategies. Key ways AI contributes to market predictions include:
- Data Analysis: AI algorithms process massive datasets far beyond human capacity, identifying patterns and correlations that might be missed otherwise.
- Predictive Modeling: Machine learning models, powered by AI, generate forecasts based on historical data, market trends, and other relevant variables.
- Real-time Analysis: AI systems provide real-time insights and updates, allowing for more dynamic and responsive trading strategies.
- Risk Management: AI helps in assessing risks by analyzing market volatility and historical performance, providing more informed decision-making tools.
Understanding P-values in Financial Market Predictions
In statistical analysis, P-values are used to determine the strength of evidence against a null hypothesis. The null hypothesis typically represents a default assumption that there is no effect or difference. In financial market predictions, understanding P-values helps in:
- Evaluating Predictive Models: P-values indicate whether the observed results are statistically significant or if they could have occurred by chance.
- Testing Hypotheses: Investors and analysts use P-values to test hypotheses about market trends, trading strategies, or the impact of certain variables on market outcomes.
- Decision Making: A low P-value (typically less than 0.05) suggests strong evidence against the null hypothesis, implying that the predictive model or trading strategy is statistically significant.
Statistical Significance and Its Importance
Statistical significance measures whether the results of an analysis are likely to be genuine rather than due to random chance. In financial markets, statistical significance is crucial for:
- Model Validation: Ensuring that AI-driven models are reliable and their predictions are not just random fluctuations.
- Risk Assessment: Determining whether the observed effects or trends are consistent and not the result of sampling variability.
- Strategic Decisions: Making informed investment decisions based on statistically significant data rather than assumptions or biases.
Challenges and Considerations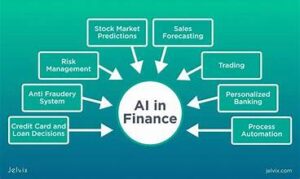
Despite its advantages, integrating AI into financial market predictions presents several challenges:
- Data Quality: AI models rely heavily on the quality of data. Inaccurate or biased data can lead to misleading predictions.
- Overfitting: AI models may overfit to historical data, reducing their effectiveness in predicting future market movements.
- Interpreting Results: Misunderstanding P-values and statistical significance can lead to incorrect conclusions and risky financial decisions.
Best Practices for Using AI in Financial Predictions
To effectively use AI in financial market predictions, consider the following best practices:
- Validate Models: Regularly test and validate AI models using out-of-sample data to ensure their predictive accuracy.
- Understand Statistical Metrics: Gain a clear understanding of P-values and statistical significance to interpret results correctly.
- Incorporate Expert Judgment: Combine AI predictions with expert financial analysis and market insights to make well-rounded decisions.
Conclusion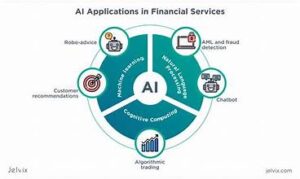
AI’s impact on financial market predictions is profound, offering powerful tools for data analysis, predictive modeling, and risk management. However, understanding statistical concepts such as P-values and statistical significance is essential to harness AI’s full potential and make informed financial decisions. By embracing best practices and staying informed about the latest advancements in AI and statistical analysis, investors and analysts can navigate the complexities of financial markets with greater confidence and accuracy.