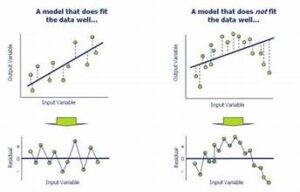
In the realm of data science, understanding statistical principles is crucial for deriving meaningful insights and making informed decisions. One such principle is the Law of Large Numbers (LLN), a foundational concept that underpins much of statistical analysis and data science methodologies. As we navigate through 2024, the LLN continues to play a pivotal role in data science, influencing everything from algorithmic predictions to risk management. This article explores the key insights and applications of the Law of Large Numbers in the context of data science, illustrating its importance and relevance in today’s data-driven world.
What is the Law of Large Numbers?
The Law of Large Numbers is a fundamental theorem in probability theory that describes the result of performing the same experiment a large number of times. It states that as the number of trials increases, the sample mean of the observed outcomes will converge to the expected value (or population mean) of the distribution.
There are two main versions of the Law of Large Numbers:
- Weak Law of Large Numbers (WLLN): This version asserts that the sample mean converges in probability to the expected value as the sample size approaches infinity. In simpler terms, with a sufficiently large sample size, the average of the observed data will be close to the expected value with high probability.
- Strong Law of Large Numbers (SLLN): This stronger version states that the sample mean will almost surely converge to the expected value as the sample size grows indefinitely. This implies that with a sufficiently large number of trials, the sample mean will almost certainly equal the population mean.
Key Insights for Data Science
- Reliability of Predictions: In data science, the LLN provides a basis for the reliability of predictions made using statistical models. By ensuring that sample estimates converge to the true population parameters, data scientists can trust that their models will perform consistently when applied to large datasets. This is particularly important in machine learning, where algorithms are often trained on large datasets to make predictions.
- Estimation Accuracy: The LLN plays a crucial role in the estimation of population parameters. For instance, when estimating the average income of a population based on a sample, the LLN ensures that as the sample size increases, the estimate will get closer to the actual average income. This principle is used in various fields such as market research, finance, and public health to make accurate estimates and forecasts.
- Decision Making: The LLN is fundamental to decision-making processes that rely on statistical evidence. For example, in A/B testing, where two versions of a product or service are compared, the LLN ensures that the results of the test will accurately reflect the true performance differences between the two versions as the sample size grows. This helps businesses make data-driven decisions with greater confidence.
- Risk Management: In finance and insurance, the LLN is used to manage and mitigate risks. By analyzing large datasets, financial analysts and actuaries can estimate the probability of rare events and set appropriate risk premiums. The LLN helps in predicting future risks based on historical data, thus aiding in the formulation of effective risk management strategies.
Applications in Data Science for 2024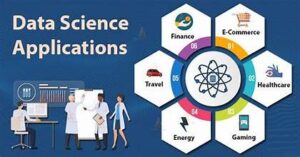
- Machine Learning and AI: The LLN is integral to the development and training of machine learning models. Algorithms that rely on large datasets, such as deep learning models, benefit from the LLN as it ensures that the training data will provide reliable insights and predictions. In 2024, advancements in machine learning continue to leverage the LLN to improve model accuracy and performance.
- Big Data Analytics: With the rise of big data, the LLN becomes increasingly relevant. Large datasets are now common in industries such as e-commerce, healthcare, and finance. The LLN helps data scientists and analysts to make sense of these massive datasets by ensuring that sample statistics reflect the true characteristics of the population.
- Predictive Modeling: Predictive modeling relies on the LLN to make accurate forecasts based on historical data. In sectors such as marketing, where predicting customer behavior is crucial, the LLN ensures that models based on large datasets will yield reliable predictions. This allows businesses to tailor their strategies and improve their targeting efforts.
- Quality Control: In manufacturing and production, the LLN is used to monitor and improve quality control processes. By analyzing large samples of production data, companies can identify defects and ensure that their products meet quality standards. The LLN ensures that quality assessments based on large samples are representative of the entire production.
- Healthcare and Medicine: In the field of healthcare, the LLN is applied to clinical trials and epidemiological studies. Large sample sizes are crucial for obtaining reliable results and making generalizations about treatment effectiveness or disease prevalence. The LLN helps ensure that these studies provide accurate and actionable insights.
Challenges and Considerations
While the LLN is a powerful tool, there are several challenges and considerations to keep in mind:
- Sample Size: While the LLN guarantees convergence to the population mean as the sample size grows, in practice, obtaining a sufficiently large sample can be challenging. Data scientists must carefully plan their studies and ensure that sample sizes are adequate to achieve reliable results.
- Data Quality: The LLN assumes that the data collected is representative and unbiased. However, issues such as measurement errors, sampling biases, and missing data can affect the validity of the results. Ensuring high-quality data is essential for the LLN to be effective.
- Computational Resources: Analyzing large datasets requires substantial computational resources. Data scientists must be prepared to handle the demands of processing and analyzing large volumes of data, which can involve significant computational and storage costs.
Conclusion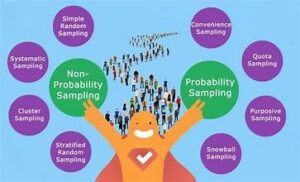
The Law of Large Numbers remains a cornerstone of statistical analysis and data science in 2024. Its principles underpin many of the techniques and methodologies used to analyze large datasets, make predictions, and manage risks. By understanding and applying the LLN, data scientists can ensure the reliability and accuracy of their analyses, leading to more informed decisions and better outcomes across various fields. As data science continues to evolve, the LLN will undoubtedly remain a key element in navigating the complexities of data and deriving meaningful insights.